Google releases Fully Homomorphic Encryption toolkit on GitHub
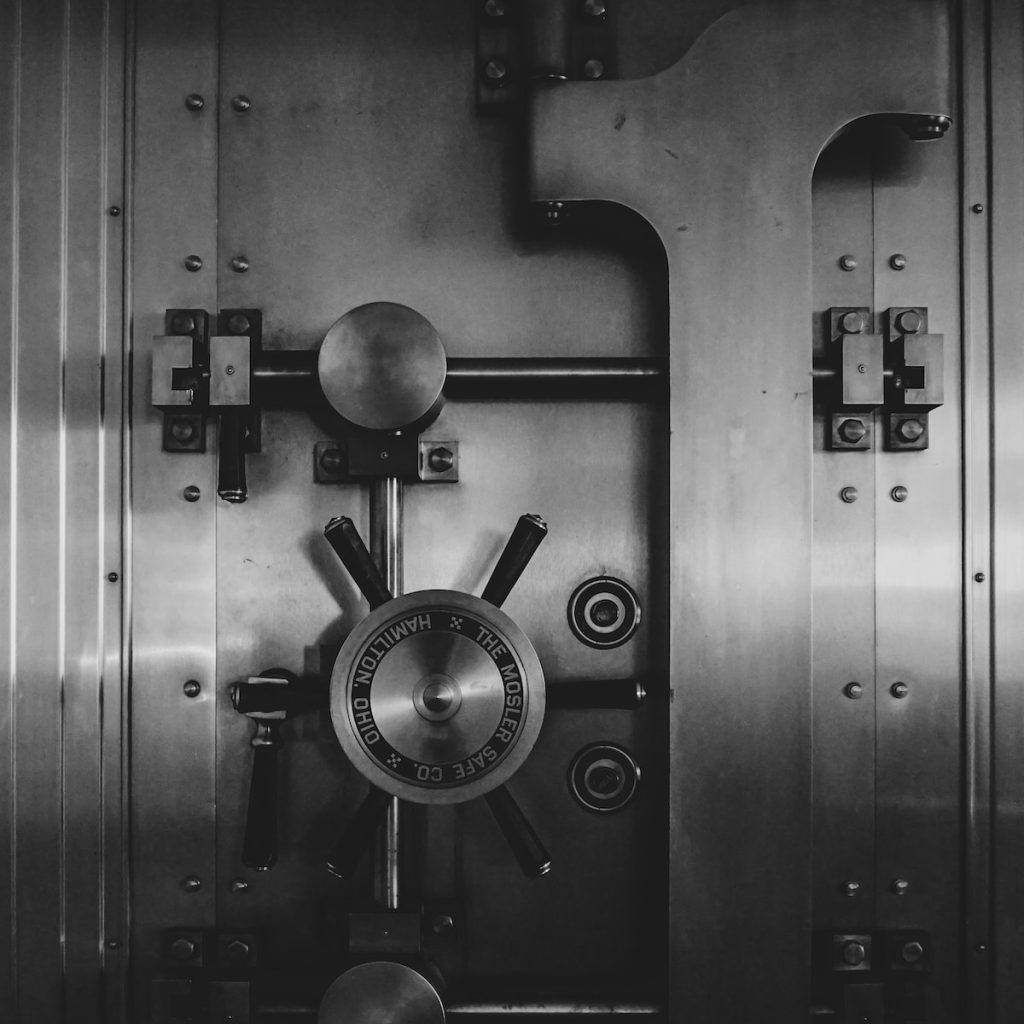
This week Google has open-sourced its Fully Homomorphic Encryption (FHE) toolkit on GitHub.
The toolkit includes open-source libraries enabling developers and data scientists to perform FHE operations on encrypted data sets:
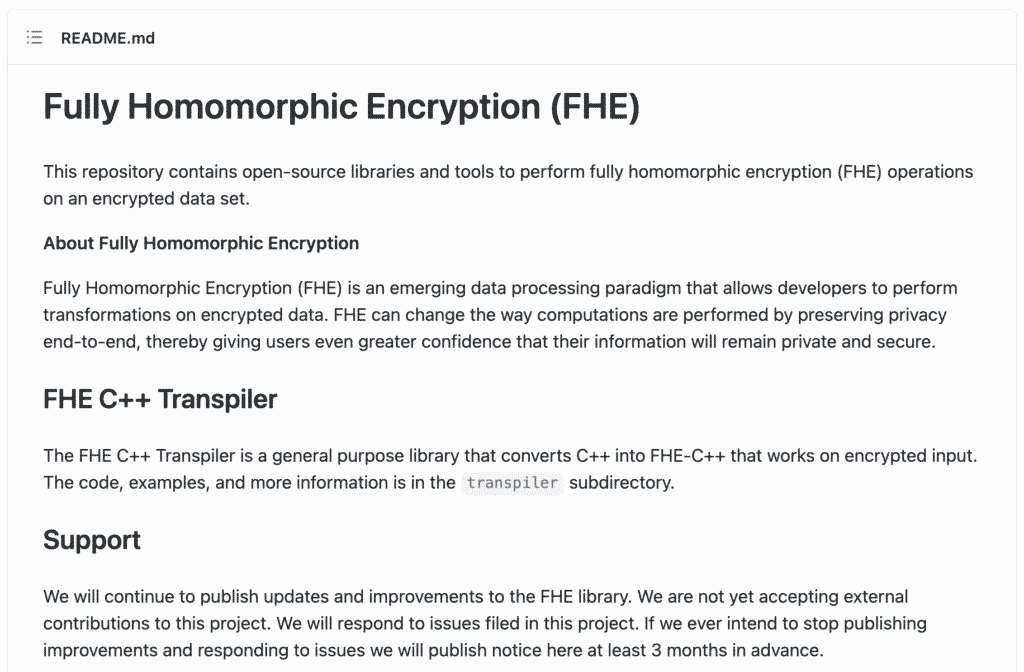
As explained in my CSO piece earlier, homomorphic encryption lets you conduct operations and computations on encrypted data without having to decrypt it and still getting reliable results:
What do you do when you need to perform computations on large data sets while preserving their confidentiality? In other words, you would like to gather analytics, for example, on user data, without revealing the contents to the computation engine that is going to calculate the analytics. Or you are a cloud services provider supporting encrypted storage of documents but want to enable your users to edit their documents without decrypting them first.
This is where homomorphic encryption could come to your rescue. Homomorphic encryption provides the ability to “outsource” the storage and computation of data to cloud environments by converting the data into an encrypted form first.
What’s noteworthy about this technique is you achieve the same results (in encrypted form) by performing the desired operations and computations on the encrypted data as you would have by performing the same operations on its unencrypted form.
The move by Google to open-source their FHE libraries is meant to encourage developers to preserve user privacy and conduct their tasks without exposing themselves to sensitive or personally identifiable information (PII).
“As developers, it’s our responsibility to help keep our users safe online and protect their data. This starts with building products that are secure by default, private by design, and put users in control,” said Miguel Guevara, Product Manager of Google’s Privacy and Data Protection Office.
Google’s FHE solution is specially useful for AI/ML developers and scientists working with healthcare data:
“FHE can also be used to train machine learning models on sensitive data in a private manner.”
“For example, imagine you’re building an application for people with diabetes. This app might collect sensitive information from its users, and you need a way to keep this data private and protected while also sharing it with medical experts to learn valuable insights that could lead to important medical advancements.”
“With Google’s transpiler for FHE, you can encrypt the data you collect and share it with medical experts who, in turn, can analyze the data without decrypting it – providing helpful information to the medical community, all while ensuring that no one can access the data’s underlying information,” further explained Guevara.
So why not go ahead and checkout the project on GitHub!